Screening for heart valve disease with acoustics and AI
Andrew McDonald, Anurag Agarwal
Valvular Heart Disease
1.5 million people in the UK suffer from significant heart valve disease.
More than half of these cases (800,000) remain undiagnosed [1]. Because of how quickly the UK population is ageing, this number will double by 2050.
Patients with late-stage valve disease have a worse prognosis than most advanced stage cancers. Early detection of the disease is essential to enable timely treatment, but clinicians can struggle to hear the disease when using a traditional stethoscope and more than half of significant cases are missed. Additionally, unnecessary referrals for benign sounds puts an immense strain on hospital cardiology departments.
We are designing a an AI-enabled acoustic screening device for valve disease. It will enable any clinician to accurately test for valvular disease, improving early detection and reducing unnecessary referrals.
Heart Sounds
The heart produces characteristic sounds, which can be heard at the chest using a stethoscope. Normal hearts produce two sounds, S1 and S2, which give a characteristic ‘lub-dub’ rhythm:
Heart disease can cause the production of murmurs – abnormal high-frequency sounds. One example is a severe aortic stenosis murmur that occurs between S1 and S2:
Machine Learning
We are applying state-of-the-art machine learning models to heart sound recordings, to identify pathological murmurs and produce an instant diagnosis of valvular heart disease.
Heart sounds are recorded from the chest in various positions, using an electronic sensor.
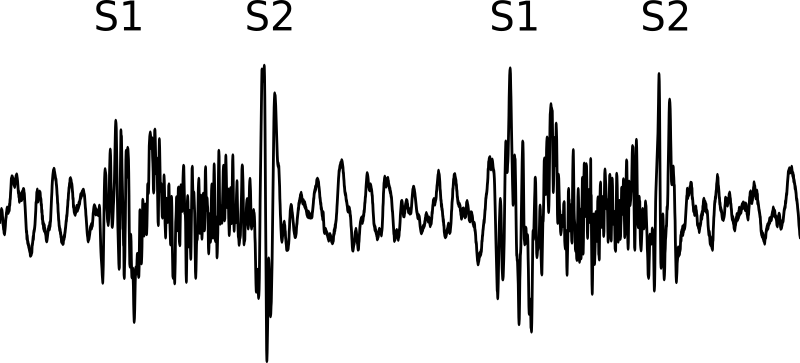
Frequency transforms applied to the heart sound separate out S1 and S2 from the higher frequency murmur.
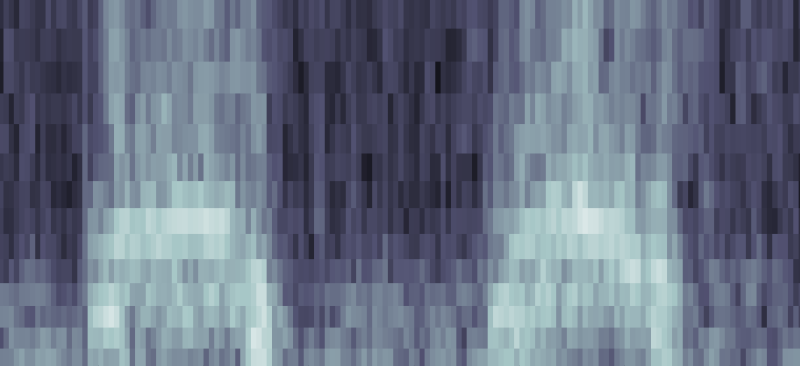
Artificial neural networks are trained to use the frequency transforms to identify the location of each heart sound, including the abnormal murmur.
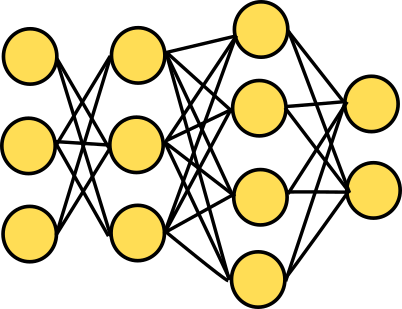
Any abnormal murmurs are reported back to the clinician for evaluation.
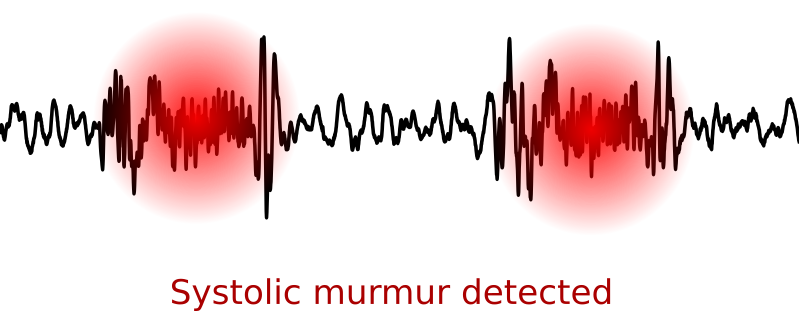
An early version of our algorithm [2] received an award at the Computing in Cardiology 2016 Conference in Vancouver. An updated version of this algorithm won First Prize at the 2022 George B. Moody PhysioNet Challenge on automated murmur detection and classification.
The project is now being commercialised through our spin-out company, Biophonics, in association with Cambridge Enterprise.
[2] E. Kay, A.Agarwal: DropConnected neural networks trained on time-frequency and inter-beat features for classifying heart sounds. Physiological Measurement, 38 (8), pp. 1645-1657, 2017.